2023 will be the year defined by a shift from hypergrowth to efficiency. Agent-based modeling will enable companies to employ both data science and their leaders’ industry expertise to ensure their businesses stay ahead in the face of unique challenges not seen for a decade and adopt AI more efficiently than competitors.
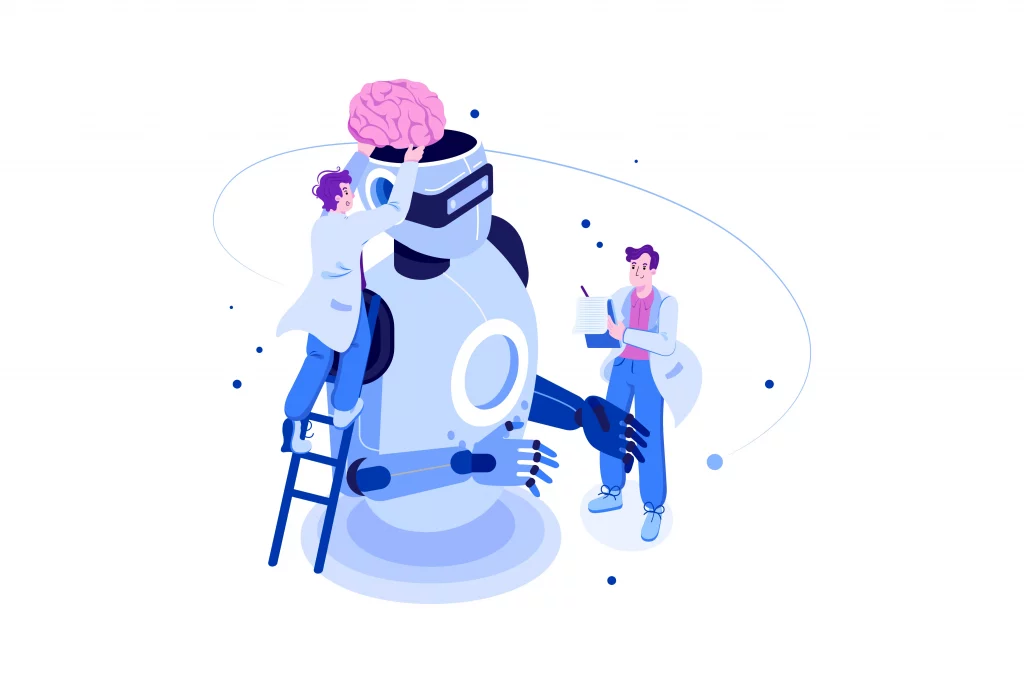
What does the AI landscape currently look like?
One clear trend of the last ten years has been a widespread investment in data analytics designed to ease the task of organizations’ decision-makers. Data science and machine learning techniques have been hailed as the “secret sauce” for driving transformation in the way companies conduct their business. Yet, outcomes have so far fallen short of expectations. According to a report by MIT and BCG, only 10% of organizations are seeing significant financial returns on their investment in AI, a clear shortfall in expectations.
What’s holding data and analytics back?
Historically, analytics has been largely data-driven, data-first. Instead of formulating problem areas and looking at how to augment data sets for an accurate answer, businesses have traditionally begun with a siloed question and looked for the right data to generate an answer.
One clear example of a failure when employing this method is the now notorious case of Google Flu Trends. In 2008, the tech giant started a service that claimed to be able to predict flu outbreaks in 25 countries. The mechanism was simple and seemingly sound: the system would perform analysis against Google flu search queries and map them across regions. It would then compare the input to a historical baseline of flu activity in that country and based on the result classify the current level as low, medium, high, or extreme.
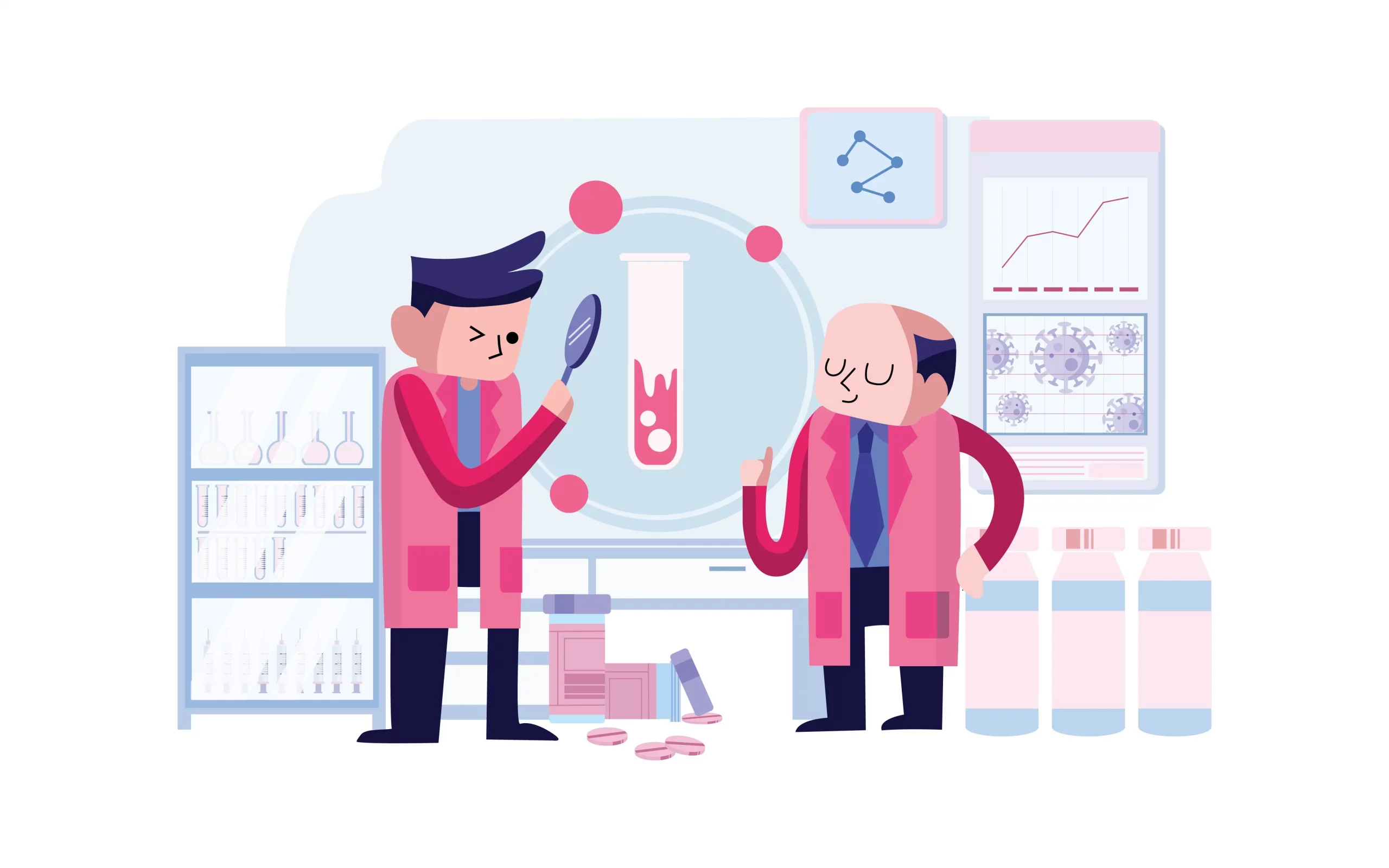
Although a viable concept, in theory, GFT’s deployment failed miserably. Simple considerations like the fact that ‘cold’ and ‘fever’ might relate to non-flu diseases or that flu symptoms vastly vary between regions and populations caused it to miss the outbreak of H1N1 in 2009 entirely. In 2013, it misestimated the number of cases at the flu peak by an astounding 140% and closed shop shortly after.
What this shortfall illustrates is that companies need to adjust from data-driven strategies to market-centric analytics. Instead of starting with data, this approach requires that organizations begin with the person and market they’re trying to understand. The trend of consumer-centric data models is also known as agent-based modeling.
Why is agent-based modeling a game changer in the way we look at data?
Organizations are turning to agent-based modeling to simulate the actions and interactions of consumers, to assess their impact on a given system. When combined with behavioral economics and network science, this is called market simulation, which allows teams to better predict the outcome of a given business action on both their brand and their competition.
The hybrid approach of business insights and numbers-based analytics addresses a reality that data alone simply cannot: As uncertain markets and economic conditions are, people are the most complex variable of all. Understanding how consumers think, feel, and make decisions — how they react in various, sometimes unexpected situations — is immensely powerful in accurately pinpointing profitable business strategies.
Looking beyond the raw data: An example
A clear illustration of why a hybrid, consumer-focused approach is much more effective, is Scios’ recent project for a B2C enterprise.
A large appliance manufacturer was faced with a variety of market dynamics that were putting their business at risk. Overseas competitors with lower-priced products were gaining market share, leading to retail store closures and steep revenue declines.
The team needed to decide whether they should adjust their pricing to respond to competitor discounts. Raw data was clearly showing pricing as the main driver for the fall in profitability and would point to lowering pricing as the key to reversing the trend.
The team used Scios Market® and found that improving online ratings and increasing sales associate recommendations would improve perceptions and boost sales in the short term. When accounting for the degree of complexity the market segment possessed, they identified that if they boosted perceptions, they could maintain a 10% price premium and gain market share from a key competitor.
Mapping out, understanding, and addressing consumer drivers allowed the manufacturer to maintain the pricing premium of its products and simultaneously gain market share from cheaper competitors.
Key Takeaways
The example in the previous section illustrates that when business strategists only focus on data related to the question instead of the entire market and what the customers’ value, the answers they get often don’t address underlying drivers. Not allowing for a degree of complexity the market possesses and therefore failing to account for the multitude of drivers when modeling that segment can lead to adverse outcomes without the need for such.
Ultimately, the most important trend for the effective adoption of AI in making strategic decisions and maximizing investment in the field for 2023 will be for business leaders to allow their models to be as complex and all-encompassing as what they’re trying to understand: their consumers.