There’s no denying AI is now the ‘cool kid on the block’ when it comes to business and strategy optimization. It received several mentions in Gartner’s Top Strategic Technology Trends in 2023 and for good reason: According to McKinsey & Company, adoption of the technology by firms has doubled since 2017, with 63% of businesses expecting increased investment in the coming years.
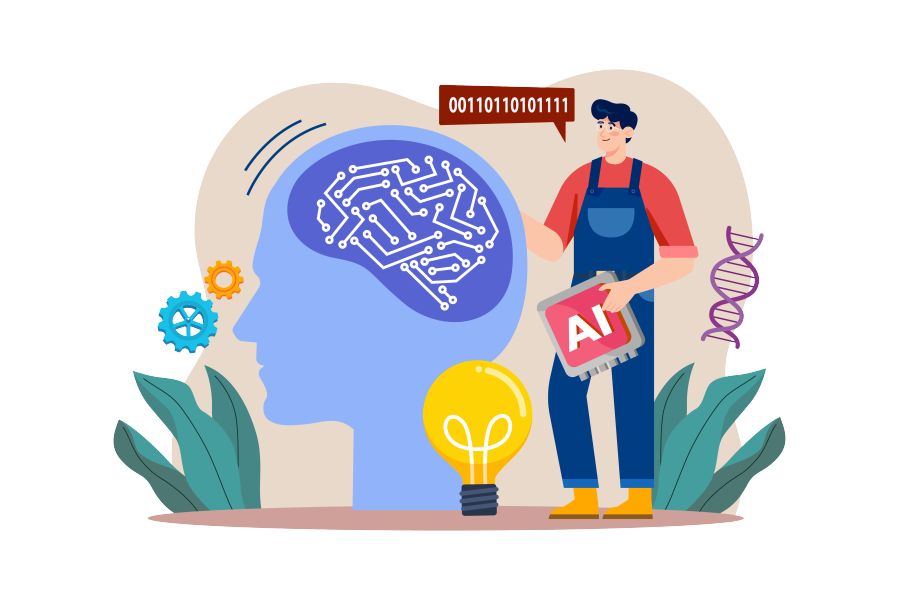
How can leaders ensure they stay ahead of the curve when it comes to advanced analytics?
Historically, the top analysis approaches have been somewhat simplistic. Simple analyses should be used to answer simple questions. However, the degree of complexity companies have to deal with has skyrocketed in recent years, boosted by global competitors and ‘Black Swan’ events like the war in Ukraine.
When complex business questions arise, there is a need for a more complex analysis that is still easy to use. This is where market simulation is gaining momentum among analytics trailblazers.
Simulation is not a new concept and has been used for decades. NASA has simulated space systems since the 1960s. The application of simulation for business purposes has started to emerge in the last decade as the prominent AI solution to test ideas in a simulated environment and predict outcomes.
Put simply, market simulation makes it possible to analyze ecosystems. By blending available data, domain expertise, and machine learning, BI teams can recreate the dynamics and rules of how a population of people in a given market behave, influence each other, and make decisions. With so many variables and rules at play, there is a level of complexity involved that is best understood through the support of AI models.
The underlying science for market simulation is rooted in agent-based modeling and enhanced with behavioral economics, network science, attribution, and machine learning.
Here is an overview of how one builds a market simulation:
Step One: Define Your Market
The first step in building a market simulation is to define the market competition. For every decision a person makes, they choose between a series of alternatives. These alternatives may include competing products, services, brands, or the choice to do nothing at all.
For example, a new TV streaming service would identify companies like Netflix, Amazon Prime Video, and Hulu as direct competitors along with cable, satellite, and premium channels. However, it’s important to also consider that a consumer may opt out of watching any programming.
Step Two: Create Simulated Consumers
Once the market is defined, then you need to identify the attributes that influence the decisions of individuals in the market and what their current preferences or perceptions are for the alternatives in the market. The simulated population may be divided into segments of consumers that have different behaviors, attitudes, and preferences. This recreates a population in a simulated environment. In order to do this, many companies use market research, segmentation studies, or other business intelligence to weigh whether people care more about price or quality, convenience, or speed.
Three: Incorporate Factors That Influence Decisions
Next, you incorporate all the other factors that influence individuals’ choices. Oftentimes, this includes the marketing activity of a particular company, competitive actions, and in some cases word-of-mouth.
Step Four: Train the Simulation
The goal of creating a simulation is that it mirrors the real-world. In order to do this, the simulation must be calibrated by adjusting settings until there is enough confidence that the simulation can reliably predict future outcomes.
This is an ongoing process since there will always be new data and learnings that need to be added to represent an evolving market. People aren’t static, so a simulation can’t be either. Over time, this allows the simulation to become smarter and even more reliable.
Step Five: Test Scenarios
With a calibrated simulation model, a company is able to begin testing insights to see how various product updates, pricing strategies, marketing campaigns, and other factors can influence consumer decision-making. Ultimately, the goal is to identify which “levers” to pull or strategies to implement in order to increase the likelihood that consumers select your product over other offerings. For example, a car manufacturer may seek to understand the impact of adding TV monitors to its line of minivans. Through market simulation, that manufacturer can clearly see the impact on sales and may decide to invest in TV monitors or pull a different lever altogether.
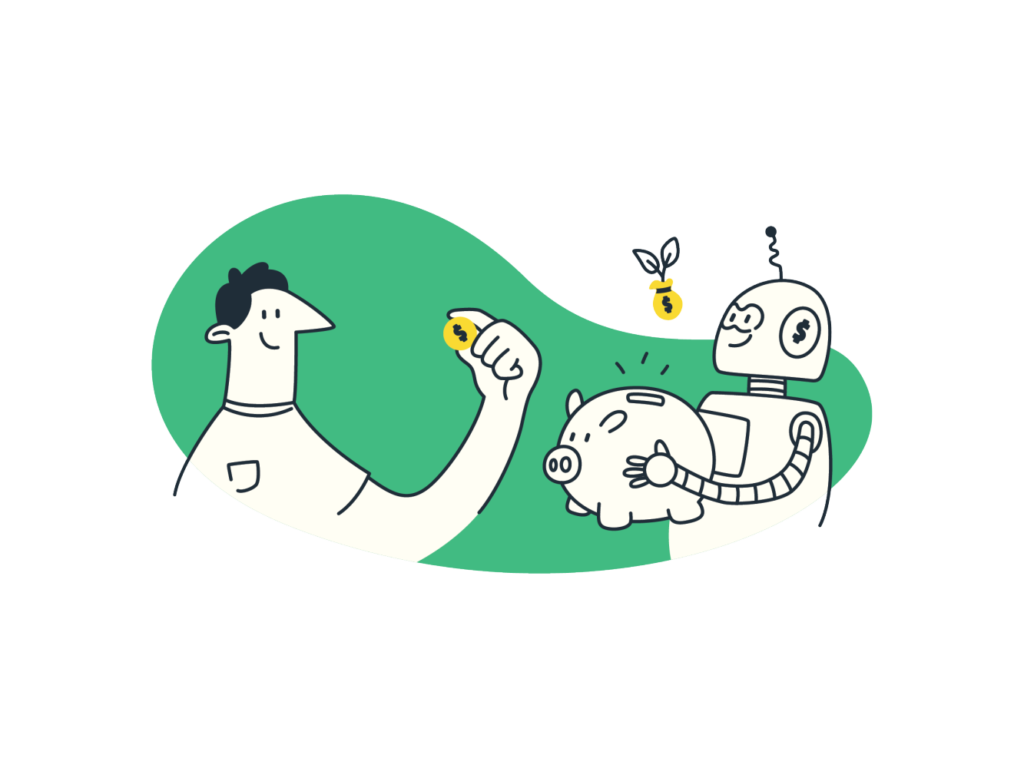
Companies are also able to leverage simulation to better understand the impact of scenarios they don’t have control over, such as a new competitSimulation is meant to build trust in data-driven insights, empowering businesses to test new strategies before making an investment. It’s the final step in realizing data as a powerful business asset that delivers ROI.or entering the market or the rise of gas prices.