In today’s data-driven landscape, organizations seek efficient ways to leverage machine learning. Enter Automated Machine Learning (AutoML), a groundbreaking solution that empowers teams to harness the full potential of their data. By automating data processing, model exploration, and tuning, AutoML streamlines the model-building process, enabling organizations to address critical business questions rapidly. From identifying customer attrition risks to predicting individual product preferences, AutoML provides the tools for success.
However, there are scenarios where machine learning’s capabilities are constrained, leading organizations to turn to market simulation. Unlike AutoML, market simulation focuses on testing what-if strategies and understanding complex real-world dynamics. It takes into account limited data, shifting dynamics, intricate interactions, and external market forces. By incorporating domain expertise, structured data, and qualitative insights, market simulation offers a broader scope for exploring interactions.
As organizations weigh their options, understanding the nuances between AutoML and market simulation becomes crucial. Here, we delve into four key considerations to help you determine the right approach for your specific needs.
Data and Expertise Requirements
AutoML thrives on the availability of extensive datasets comprising thousands, millions, or more data points. By analyzing these vast datasets, AutoML uncovers valuable patterns and insights. While domain knowledge can aid in the interpretation and validation of model outcomes, it is not a prerequisite for achieving accurate results.
In contrast, market simulation necessitates a comprehensive understanding of the functioning of a specific market. Domain knowledge plays a pivotal role right from the outset of the simulation process. This provides an opportunity for business stakeholders to actively engage and contribute their expertise, instilling a sense of confidence in the final results, as they align with their human experience.
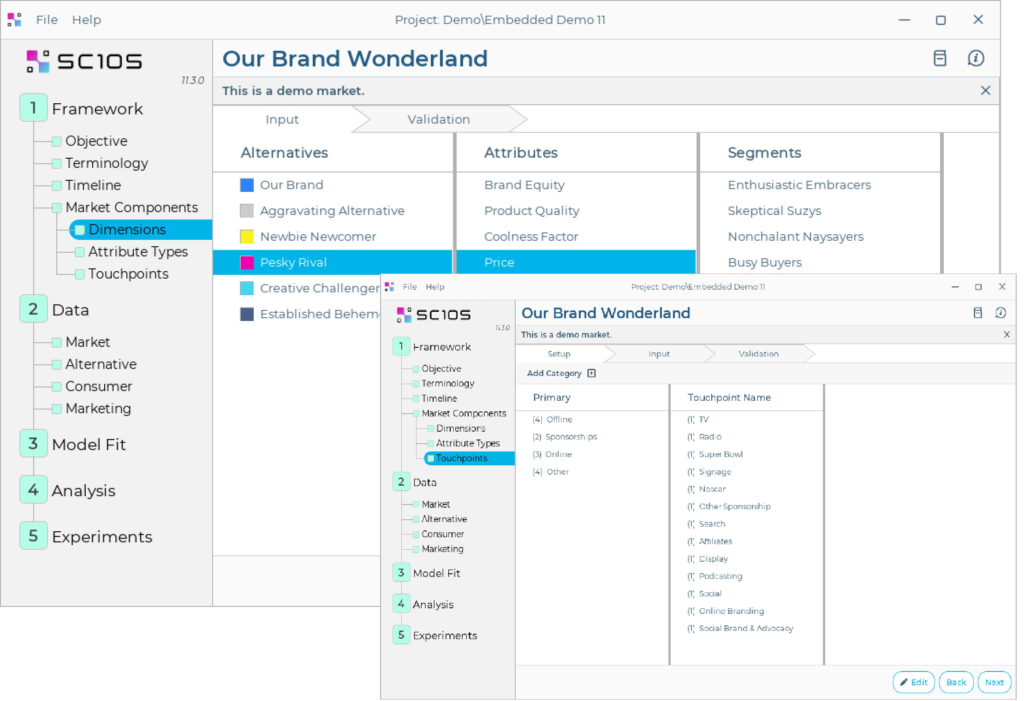
Interpretability
AutoML harnesses the power of extensive data and employs intricate algorithms to unveil hidden relationships. Its strength lies in uncovering valuable connections buried within large datasets. However, understanding the underlying reasons behind these relationships can often pose a significant challenge. The transparency of the relationships identified by AutoML may be limited, requiring data scientists to invest considerable effort in extracting and translating these insights for business users.
In contrast, market simulation delves into the construction and modeling of complex dynamics and interactions. Through the calibration process, it becomes evident which factors and levers in the market drive specific outcomes. This clarity allows for various diagnostic approaches, including sensitivity analyses and attribution analyses, enabling visualization of the key drivers behind the observed outcomes.
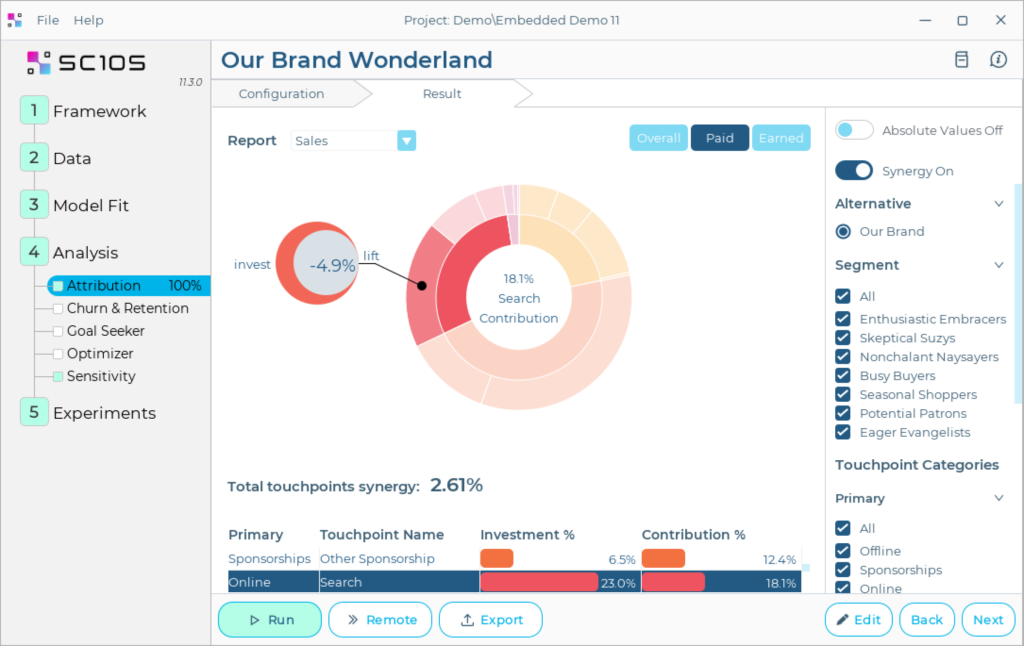
Shifting Consumer Dynamics
When armed with the right data, AutoML empowers teams to address specific tactical questions surrounding consumer preferences. For instance, by developing algorithms, teams can rank products based on their likelihood to resonate with individual customers, considering various attributes. This approach is effective as long as the underlying preferences remain stable—the status quo prevails. Thus, AutoML proves invaluable in managing short-term, tactical decisions.
On the other hand, market simulation excels in navigating the ever-changing landscape of consumer preferences. For instance, consumer behavior may drastically vary before, during, and after significant events like brand campaigns, recessions, or PR crises. By incorporating data and insights on consumer perceptions and drivers of choice, market simulation unveils how these events and interactions shape the evolution of consumer preferences over time. Market simulation empowers teams to make strategic choices amidst shifting economic environments and consumer trends. Moreover, it proves invaluable when conducting what-if forecasting for future changes on the horizon.
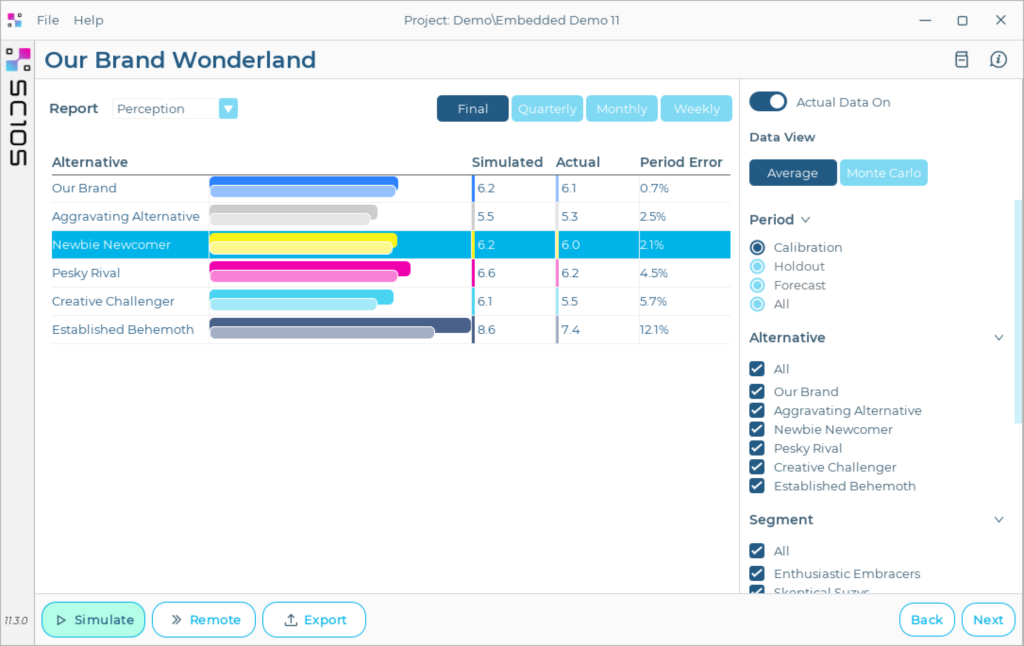
Competition
AutoML proves valuable in modeling behavioral patterns of individual consumers, offering insights into specific questions such as identifying at-risk customers or predicting personalized TV program preferences. It guides tactics related to customer retention and personalization, enabling organizations to make targeted decisions.
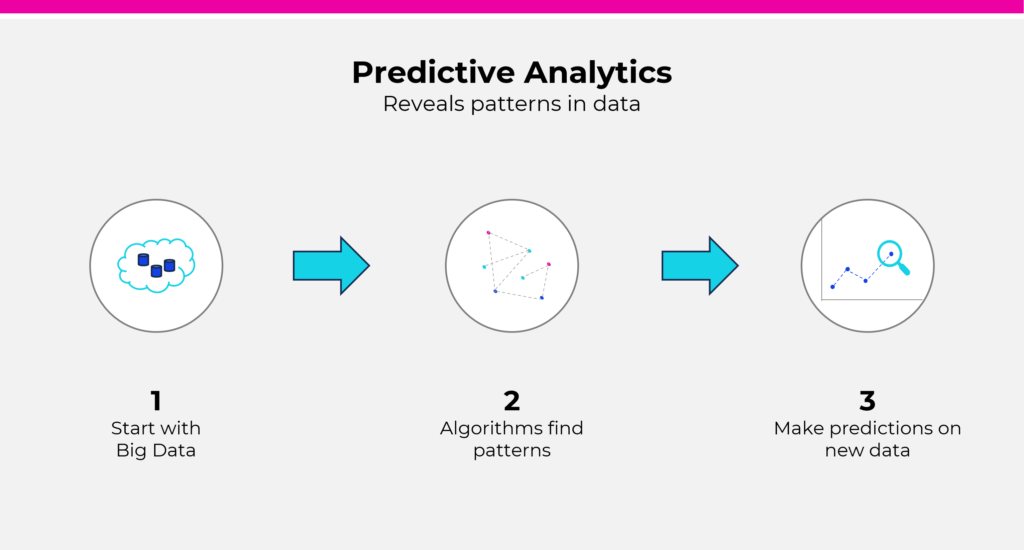
In contrast, market simulation focuses on understanding population behavior and modeling how consumers make choices among competing alternatives. By placing their brand within the competitive landscape, teams can leverage market simulation to explore what-if scenarios and address strategic questions. This capability empowers organizations to navigate competitive market strategies, such as assessing the impact of their business strategy on market share, evaluating the influence of competitor strategies on market outcomes, and determining the optimal response to competitive shifts that would benefit their brand.
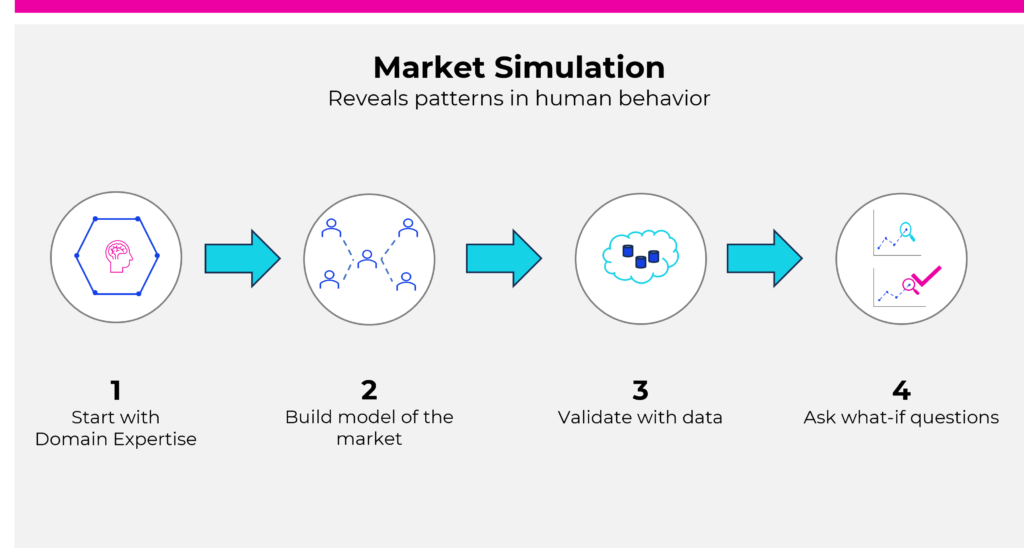
Managing the forest and the trees
A helpful analogy when comparing strategic planning to tactical execution is thinking of the forest (the broader business strategy) and the trees (specific tactical questions). In this context, market simulation emerges as a catalyst, empowering organizations to leverage their data and expertise to inform strategic decision-making through what-if scenario testing. AutoML acts as a valuable complement to market simulation, offering tactical insights for specific problem-solving scenarios where abundant structured data is available.
By embracing both market simulation and AutoML, teams gain a comprehensive toolset that enables effective management of both the forest (strategic decisions) and the trees (specific tactical challenges). This holistic approach empowers organizations to strike a balance between long-term strategic vision and short-term execution, leading to informed and impactful business outcomes.